Stable Cascade | Text to Image
In this ComfyUI workflow, we use Stable Cascade, a text-to-image model that performs better in both prompt alignment and aesthetic quality in almost all model comparisons. You can try a more detailed prompt to see the result.ComfyUI Stable Cascade Workflow
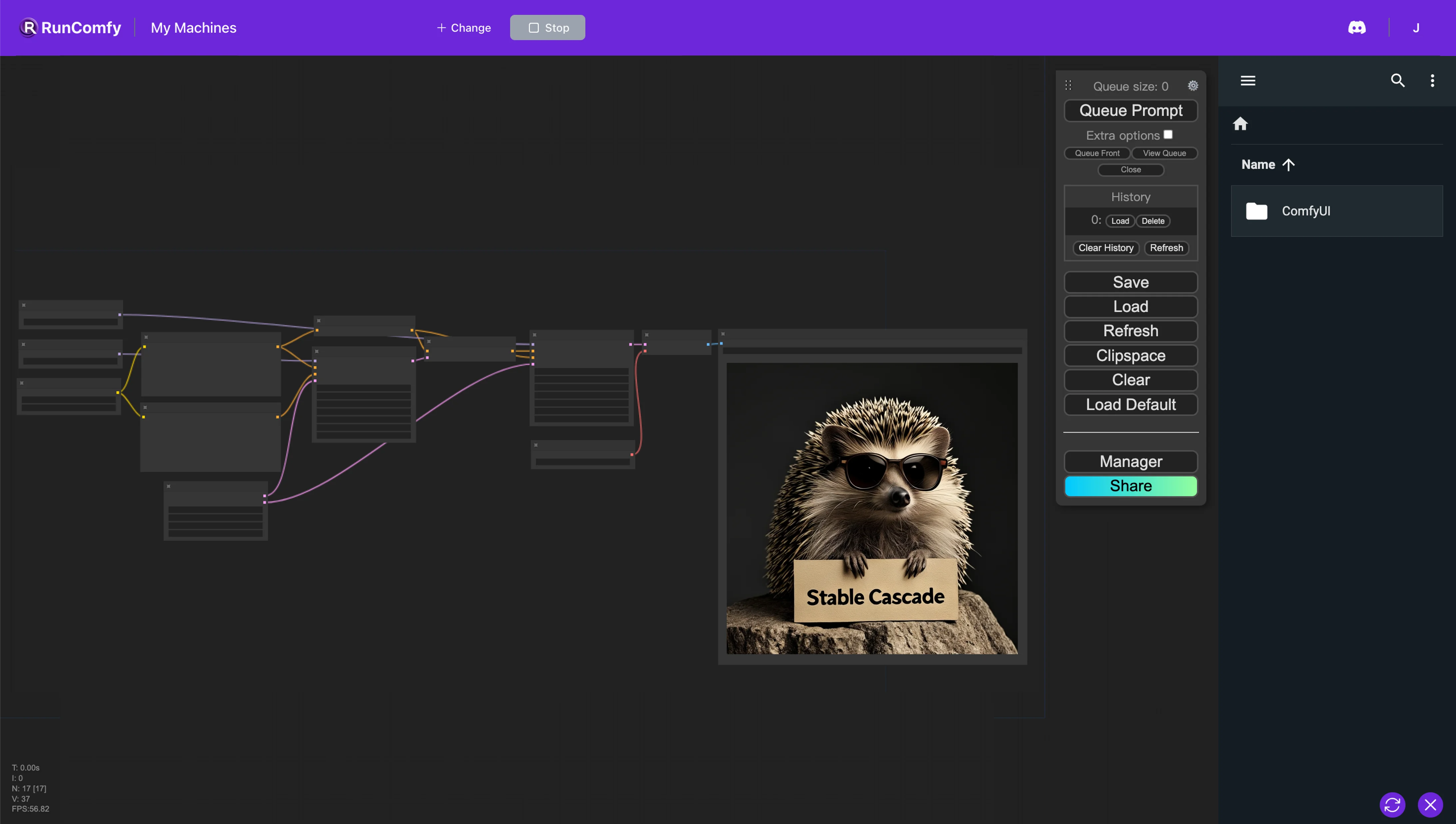
- Fully operational workflows
- No missing nodes or models
- No manual setups required
- Features stunning visuals
ComfyUI Stable Cascade Examples
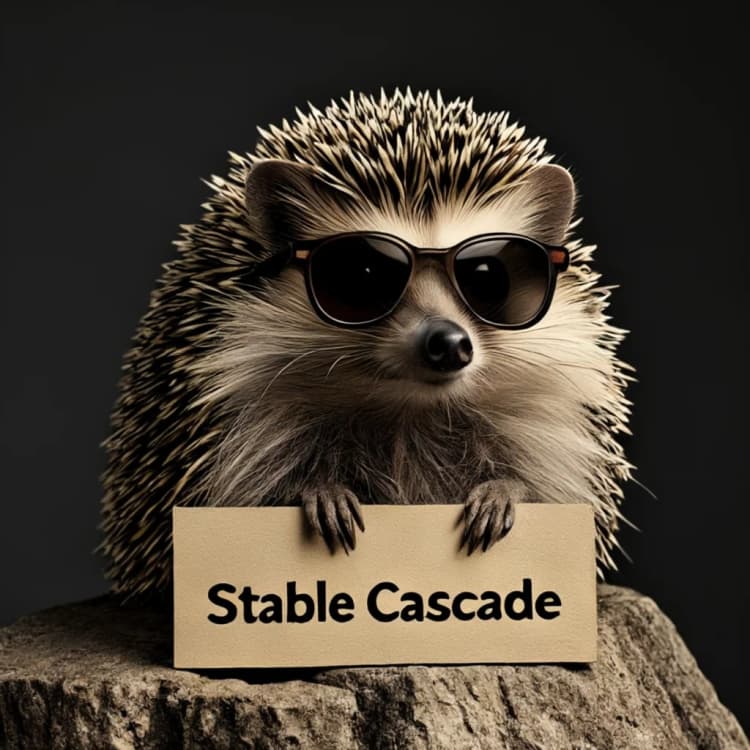
ComfyUI Stable Cascade Description
1. Stable Cascade ComfyUI Workflow
In this ComfyUI workflow, we leverage Stable Cascade, a superior text-to-image model noted for its prompt alignment and aesthetic excellence. Unlike other Stable Diffusion models, Stable Cascade utilizes a three-stage pipeline (Stages A, B, and C) architecture. This design enables hierarchical image compression in a highly efficient latent space, resulting in exceptional image quality.
2. Overview of Stable Cascade
Stable Cascade emerges as a groundbreaking text-to-image model, leveraging the innovative architecture. This model distinguishes itself through its higher quality images, faster speeds, lower costs, and easier customization.
2.1. A Three-Stage Process Structure
Stable Cascade Stage A: Stage A of Stable Cascade utilizes a Vector-Quantized Generative Adversarial Network (VQGAN) to achieve image compression by a factor of four. This stage innovatively quantizes values into one of 8,192 unique entries from a learned codebook, akin to selecting colors from a palette. This quantization not only spatially compresses the image 4:1 but also significantly reduces the data size by representing images with discrete tokens. This method stands in contrast to Stable Diffusion's use of floating point values, offering a more compact and efficient compression technique.
Stable Cascade Stage B: Moving forward to Stage B, Stable Cascade showcases its prowess in refining image data. Here, the discrete tokens from Stage A undergo transformation through a latent diffusion model, ingeniously integrating the principles of an IP Adapter with diffusion techniques to guide the creation of similar output images. Stage B shines in its ability to transform tokenized data back into rich, detailed floating-point values, enhancing the image's semantic quality. This stage is designed for efficiency, focusing on creating denoised latents that perfectly match the input, thereby making the training process more streamlined and reducing computational demands.
Stable Cascade Stage C: Stage C introduces a novel approach by adding noise to the semantic output from Stage B, then meticulously denoising it using a sequence of ConvNeXt blocks. The aim is to precisely replicate the semantic content, bypassing the need for downsampling. This stage plays a pivotal role in transforming a semantic blob into a coherent piece that Stage B can further refine, culminating in the generation of high-quality images. Stage C's strategic use of ConvNeXt blocks highlights its commitment to delivering top-notch performance efficiently, sidestepping the hefty computational costs typically involved in achieving such advanced results.
2.2. Why Stable Cascade Stands Out
Superior Aesthetic Quality: Evaluations reveal that Stable Cascade significantly surpasses Stable Diffusion XL in delivering visually stunning images. It achieves 2.5 times the aesthetic quality of SDXL and astonishingly outperforms SDXL Turbo by 5.5 times, showcasing its exceptional capability in producing high-quality visuals.
Enhanced Inference Speed: Thanks to its innovative architecture, Stable Cascade offers a more efficient inference process, utilizing resources more effectively than its predecessors. With a remarkable compression factor of 42, it can transform 1024x1024 images into compact 24x24 dimensions. This efficiency does not compromise image quality but rather speeds up the generation process, making it a game-changer for generating images quickly.
Improved Prompt Understanding: Stable Cascade also shines in its ability to understand and align with user prompts, whether they are brief or detailed. Human evaluations have demonstrated that it outperforms other models in accurately interpreting prompts, ensuring that the generated images closely match the user's vision.